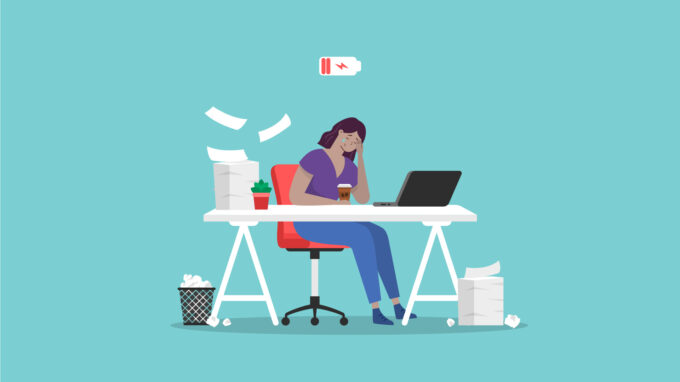
Can We Avoid Burnout?
We often use the term “burnout” as a vague label for an exhausting day or week of hard work, but actual burnout is more than
For decades, futurists have been predicting that the workplace will one day be radically transformed by artificial intelligence (AI) and machine learning.
But it’s only fairly recently that the technology has begun to demonstrate its potential. AI and machine learning technologies are becoming more and more accessible and affordable thanks to the exponential growth in computing power, the development of more and more sophisticated algorithms, and the massive amounts of data generated by billions of people every day.
“One day” is here.
Parts of the financial sector, such as mortgage approvals, have been automated for years. Chatbots and virtual assistants are taking over many customer service functions traditionally handled by people. Automation in factories has eliminated jobs in some areas while creating employment in others.
While AI and machine learning is benefiting businesses and organizations in every sector, the inevitable disruption also brings major challenges. Too often, the conversation focuses on the extremes: whether human workers will become obsolete, or that AI will relieve people of repetitive or dangerous tasks and increase profits while allowing humans to pursue more creative pursuits. Organizations need to shift that conversation.
The difference between success and failure will depend on how well leaders prepare their workforces for a world where employees work with — rather than compete against— AI, machine learning, robots and other “smart” technologies.
What do we mean by AI and machine learning? People often conflate the two concepts but there are key differences.
In a nutshell, machine learning is the use of mathematical procedures (algorithms) to analyze and find patterns in data which can then be used to make predictions about the world. For example, retail stores use machine learning to analyze customers’ purchasing history in order to predict which products will be in demand and make appropriate decisions about things like inventory or pricing. As more and more quality data are made available to the algorithm, the greater the reliability of its predictions. The algorithm “learns” without requiring the intervention of a computer programmer to tell it what to do next.
It’s a lot like how people learn. You observe what goes on around you, drawing conclusions and identifying patterns about how the world works. You then use this knowledge to deal with new situations as they arise. Machine learning’s advantage over human learning is its ability to process much more data, to deploy more sophisticated algorithms to identify patterns, and to do this at great speed. This leads to fast, reliable predictions that can exceed human decision-making capabilities and mitigate unconscious bias (which we will discuss in more detail below).
Machine learning is a subset of AI. AI is the replication of human analytical and decision-making capabilities through the application of sophisticated machine learning. There are AI applications that can perform as well or better than humans at a variety of tasks, from playing chess to assessing creditworthiness to spotting a cancerous tumour on an x-ray. AI is a form of intelligence, but it is not conscious in the way that human minds are.
Machine learning has been used in many industries for years. Most people engage with it in some form every day. It’s used for fraud detection and credit scoring, placing real-time ads on mobile devices and websites, web search results, spam filtering, image and pattern recognition, sentiment analysis in texts, and more. Machine learning provides the “brains” behind everything from pizza-making robots to self-driving cars to computer programs that write articles for the sports and finance pages. Sectors that are leading the way include:
It’s been estimated that in many fields, predictive models can outperform human decision makers by 20 to 30 per cent. Leveraging machine learning and AI can help make your workforce more efficient and deliver valuable business intelligence to improve your value proposition, customer service, logistics, marketing efforts, and more.
But simply putting more data in the hands of employees will not, by itself, translate into improved business performance. A successful transition will depend on the ability to create a machine learning-ready workplace culture. In practice, this means that in addition to investing in the latest technology, companies will also need to invest in training and enhancing the skillsets of their employees.
That’s because, in the end, machine learning is just math. It is only a tool, and like all tools, algorithms are only as good as the people who design them and use them, and the materials — the data — that they’re given to work with. Ultimately, it will be a human’s job to ensure that the data being collected are relevant and that the insights it shows are being leveraged properly.
So how do you position your workforce to effectively carry out the crucial role of making decisions and acting on the insights from machine learning algorithms?
You need to build a culture that values critical thinking.
Critical thinking is the ability reflect upon information in order to form good judgments and actions. To borrow from the algorithm analogy, a good critical thinker takes information as input, and outputs good judgments and actions. In this case, the critical thinker takes the insights and predictions produced by machine learning algorithms as inputs, and outputs sound judgments and actions that will move the organization forward.
Leaders need to develop the capacity for critical thinking across their organization so that as machine learning gets more integrated into everyone’s work, the organization as a whole is making the best decisions it can.
Machine learning is really good at making predictions about a very narrow set of things. It is a ‘vertical integrator’ of information — or, it has ‘vertical intelligence.’ Its intelligence is narrow and deep (relative to humans). Humans are really good at integrating a wide set of information. We’re horizontal integrators. Human intelligence is wide and shallow (relative to algorithms).
Since business decisions don’t happen in a vacuum, organizations need to leverage humans’ ability to integrate machine learning insights into the wider organizational and business context to make good decisions — and to avoid bad ones. For example, there are many algorithm-based market intelligence tools than can help businesses understand their customers and develop messages that will resonate with them. However, if the winning formula predicted by the algorithm is, say, already being used by one of your competitors, or is based on a small or suspect data set, you wouldn’t run with these messages because to do so could cause confusion and even damage your brand. Human intelligence and creativity are still required to fine-tune messages that connect with the right audience at the right time.
Understanding the larger context helps us identify when algorithms produce suboptimal results. Recently, Amazon abandoned its AI-powered recruitment engine when it was discovered to have a built-in bias against hiring women. That’s because the computer models used to vet applicants were based on resumes submitted to the company over the previous decade. Most of the job applicants in that period were men — a reflection of male dominance in the tech industry. Amazon’s system taught itself to prefer male over female candidates, but the machine wasn’t biased — the data set was.
So, machine learning isn’t magic — it depends on people asking the right questions to solve the right problems in the first place, and interpreting machine learning insights in a human real-world context. To make all that happen, leaders must equip their employees with three critical thinking abilities: the critical thinking mindset, skill set and tool set.
The core of critical thinking is really an attitude or mindset that comes down to a very simple behaviour: the ability to consider an idea without necessarily accepting that idea as true.
When you can entertain a thought without automatically accepting it, you are able to open up a space between understanding something and acting on it. And that’s precisely what you need from your workforce in order to reduce your risk-exposure when integrating more machine learning technologies: your workforce needs to be able to reflect on machine learning algorithms and insights before blindly putting them into practice.
The following behaviours demonstrate when an individual has adopted a critical thinking mindset:
We know it is possible for algorithms to be biased, as the Amazon example above makes clear.
The reason they can be biased is an example of the “garbage in, garbage out” principle. Machine learning algorithms learn — without reflection — from data sets. So if the data sets are biased in some way — say, by containing more men than women — then the predictions that the data sets deliver will be biased in the same way.
That’s why having some checks and balances on machine learning is important. And humans armed with critical thinking skills are well-suited to this task because of our horizontal intelligence.
One way to enable your team’s critical thinking skill sets is through Pearson’s RED Critical Thinking Model. Based on a widely used test to evaluate cognitive ability, the RED model emphasizes that to think critically, we must:
Workers equipped with critical thinking skills can put machine learning insights to the test to see if they make sense — both in relation to the set of constraints imposed on the algorithm and to the broader context of the business or organization.
Building employees’ confidence in their critical thinking abilities will empower them to incorporate machine learning insights into their decision-making. In turn, that will drive innovation.
Organizations can help their people build their confidence by providing them with decision-making tools and job-aids such as this simple seven-step process.
Importantly, the tools you select should guide people’s thinking in ways that avoid unconscious biases. For that reason these tools should be located in the workplace environment (so they are external to the individual and therefore not subject to individual biases) and they should provide a common process that everyone can agree upon to deliver results that everyone can trust.
There are three kinds of tools and processes we recommend as a starting point:
Machine learning and AI can deliver competitive advantages but only if the entire organization is committed and open to change, from the CEO and top IT administrators to mid-level managers and frontline staff.
Companies that invest in equipping employees with critical thinking skills to guide this never-ending process of analyzing data, evaluating insights, making predictions, measuring results and making the adjustments will achieve better business results, increase productivity and continue to drive innovation.
About Dialectic
Dialectic provides education and tools to help people transition to a partnership with the AI-enabled robot that may be in their future. We focus on the psychological, social, emotional, and cognitive needs of humans in that partnership, and how companies can help people adapt to that world through training and tool development. Contact us to learn more.
We often use the term “burnout” as a vague label for an exhausting day or week of hard work, but actual burnout is more than
At Dialectic, we think a lot about DEI, soft skills, and leadership training that makes organizations more inclusive and human-friendly. When we team up with
Ageism commonly affects women over 40 in the workplace. In spite of their tremendous wisdom and experience, women over 40 may be passed over for
Dialectic helps organizations improve the way people work, learn, and collaborate through person-centred design and the latest in social science.
Does your team struggle with soft skills?
Use our app or upload Snippets to your LMS to build better habits in minutes with scenario-based microlearning.
Sign up for our weekly roundup of the latest on DEI, leadership, collaboration, and learning science.
© 2024 Dialectic. All rights reserved. | Contact Us | Privacy Policy | Terms of Use | AODA Statement
See how easy it is to activate soft skills in your organization. Soft skills training on 3 key topics: DEI, Leadership, and Collaboration.